Machine Learning Still Immature Despite Standardization of Ideas
Machine learning (ML) has been growing rapidly over recent years but experts have noticed something strange - despite many standard techniques becoming commonplace, they're still being ferociously cited by researchers which suggests that ML is still immature compared with other established sciences.
Dec. 24, 2022 3:06AM
Generated in 22.0 seconds
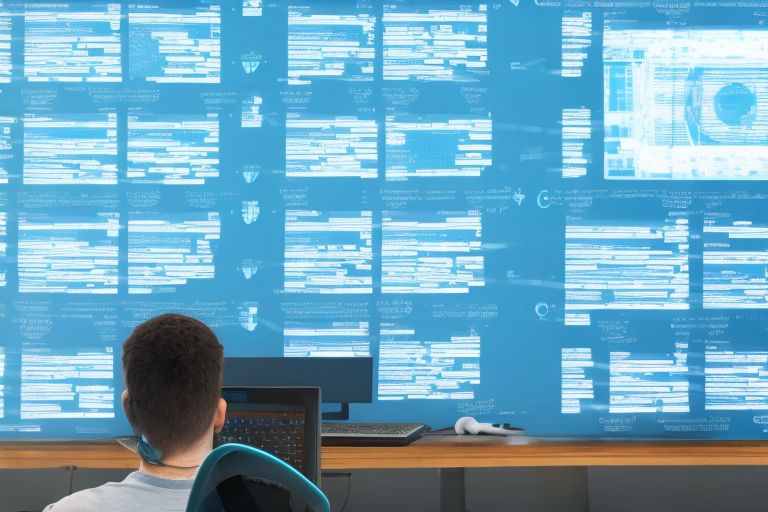
A computer monitor displaying code with various scientific equations scattered around it against a blue background with a person looking at it intently in the foreground
In the world of science, it is not uncommon for ideas to become cornerstones or be used routinely and taken for granted. This is especially true in established areas of science where these ideas are no longer cited. However, when it comes to machine learning (ML), the most standard and commoditized ideas are still being ferociously cited. This is a sign that ML is still immature according to experts in the field. The idea was brought up by an AI researcher on Twitter who pointed out this discrepancy between established sciences and ML. They suggested that this could be due to the fact that ML is a relatively new field compared to other sciences, so its ideas have yet to become accepted as the norm or taken for granted. Additionally, many of these standard ML techniques are still being improved upon with new research coming out all the time which could explain why they’re still being cited so much. However, some experts believe that this phenomenon may also be due to how competitive and cutthroat the industry has become. With more people entering into ML research than ever before, there’s a lot of pressure for researchers to make sure their work stands out from others in order to get published or receive recognition from their peers. This could lead them to cite even standard techniques more often than necessary in order to make sure their work gets noticed by others in the field. Overall, it seems like this discrepancy between established sciences and ML can be attributed both to its relative immaturity as well as its competitive nature within academia and industry alike. It will be interesting to see if this trend continues over time or if eventually these standard techniques will become taken for granted just like they have been in other areas of science.