SnowflakeDB and Apache Spark Dataframes Now Supported Out of the Box
SnowflakeDB and Apache Spark have announced that their respective dataframes are now supported out of the box, making it easier than ever before for developers who need an easy way to work with large datasets using Python or SQL languages without having to write code from scratch every time they want run queries or analyze multiple datasets at once!
Jan. 12, 2023 11:05AM
Generated in 22.9 seconds
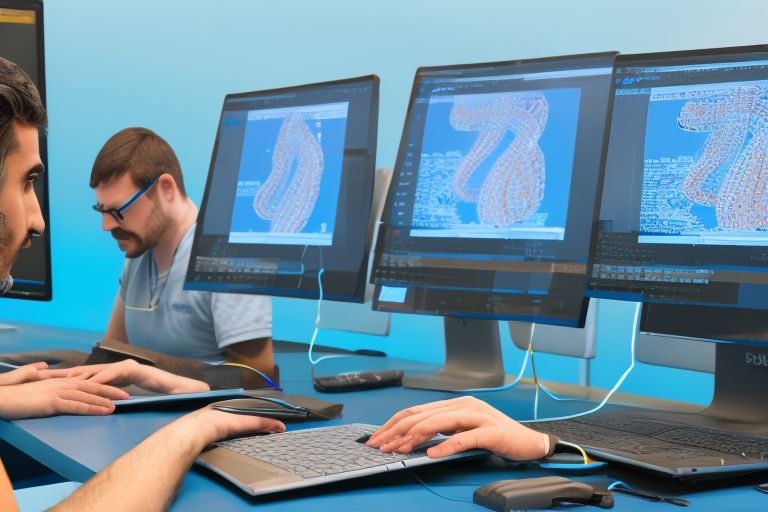
A photo showing two people working on laptops side by side while looking at a computer screen displaying code written in Python language against a blue background
In a major development, SnowflakeDB and Apache Spark have announced that their dataframes are now supported out of the box. This means that users can now pass them into any command that accepts pandas dataframes. The announcement was made on Twitter, with a link to the changelog and demo. According to the tweet, this move will make it easier for developers to work with large datasets in Python, SQL, and other programming languages. SnowflakeDB is a cloud-based data warehouse service designed for analytics workloads. It allows users to store and analyze large amounts of structured data in an efficient manner. Apache Spark is an open-source distributed computing framework used for big data processing tasks such as machine learning and streaming analytics. Both services offer powerful tools for working with large datasets. The ability to pass SnowflakeDB's Snowpark and Apache Spark's PySpark dataframes into commands that accept pandas dataframes will make it much easier for developers to work with these services. This could be especially useful when dealing with complex queries or analyzing multiple datasets at once. It also makes it simpler to integrate existing applications into new ones using these frameworks. In addition, this move could help speed up development times by reducing the amount of time needed to write code from scratch when working with large datasets in Python or SQL languages. This could result in faster turnaround times for projects involving big data analysis or machine learning tasks, allowing companies to get results faster than ever before. Overall, this announcement is sure to be welcomed by developers who need an easy way to work with large datasets using Python or SQL languages without having to write code from scratch every time they want to run a query or analyze multiple datasets at once. With this new feature, they can do all of this quickly and easily out of the box!